Harmonizing AI Innovation with Sustainable Data Center Management
Explore how AI innovation and sustainable data center management intersect, focusing on energy-efficient strategies to balance performance and environmental impact.
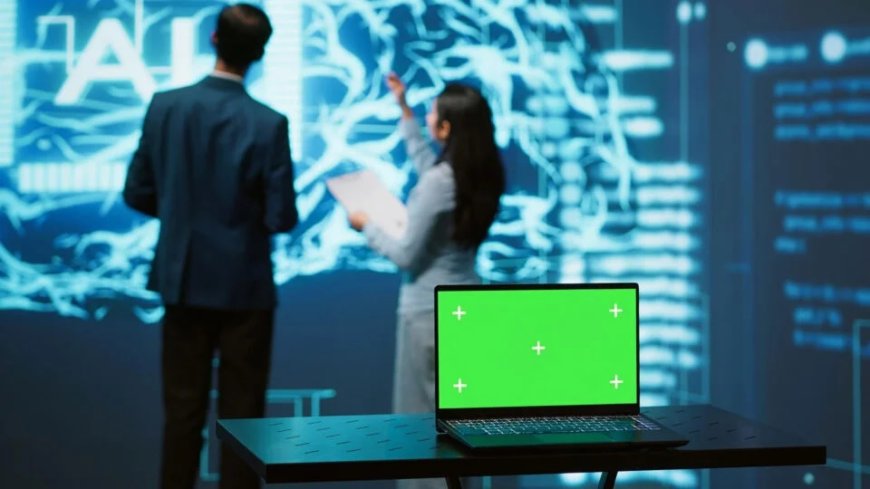
With all that’s being said about the growth in demand for AI, it’s no surprise that the topics of powering all that AI infrastructure and eking out every ounce of efficiency from these multi-million-dollar deployments are hot on the minds of those running the systems. Each data center, be it a complete facility or a floor or room in a multi-use facility, has a power budget. The question is how to get the most out of that power budget?
Key Challenges in Managing Power Consumption of AI Models
High Energy Demand: AI models, especially deep learning networks, require substantial computational power for training and inference, predominantly handled by GPUs. These GPUs consume large amounts of electricity, significantly increasing the overall energy demands on data centers. AI and machine learning workloads are reported to double computing power needs every six months. The continuous operation of AI models, processing vast amounts of data around the clock, exacerbates this issue, increasing both operational costs and energy consumption. Remember, it’s not just model training, but also inferencing and model experimentation which consume power and computing resources.
Cooling Requirements: With great power comes great heat. In addition to the total power demand increasing, the power density (i.e. kW/rack) is climbing rapidly, necessitating innovative and efficient cooling systems to maintain optimal operating temperatures. Cooling systems themselves consume a significant portion of the energy, with the International Energy Agency reporting that cooling consumed as much energy as the computing! Each function accounted for 40% of data center electricity demand with the remaining 20% from other equipment.
Scalability and Efficiency: Scaling AI applications increases the need for more computational resources, memory, and data storage, leading to higher energy consumption. Efficiently scaling AI infrastructure while keeping energy use in check is complex. Processor performance has grown faster than memory and storage’s ability to feed the processors, leading to the “Memory Wall” as a barrier to deriving high utilization of the processors’ capabilities. Unless the memory wall can be broken, users are left with a sub-optimal deployment of many under-utilized, power-eating GPUs to do the work.
In conclusion, balancing AI-driven innovation with sustainability requires a multifaceted approach, leveraging advanced technologies like computational storage drives, distributed computing, and expanded memory via CXL. These solutions can significantly reduce the energy consumption of AI infrastructure while maintaining high performance and operational efficiency. By addressing the challenges associated with power consumption and adopting innovative storage and processing technologies, data centers can achieve their sustainability goals and support the growing demands of AI and ML applications.
To Know More, Read Full Article @ https://ai-techpark.com/balancing-brains-and-brawn/
Related Articles -
AI in Drug Discovery and Material Science
Top Five Best AI Coding Assistant Tools
Trending Category - Patient Engagement/Monitoring