Big Data Analytics Solutions for Predictive Analytics and Forecasting
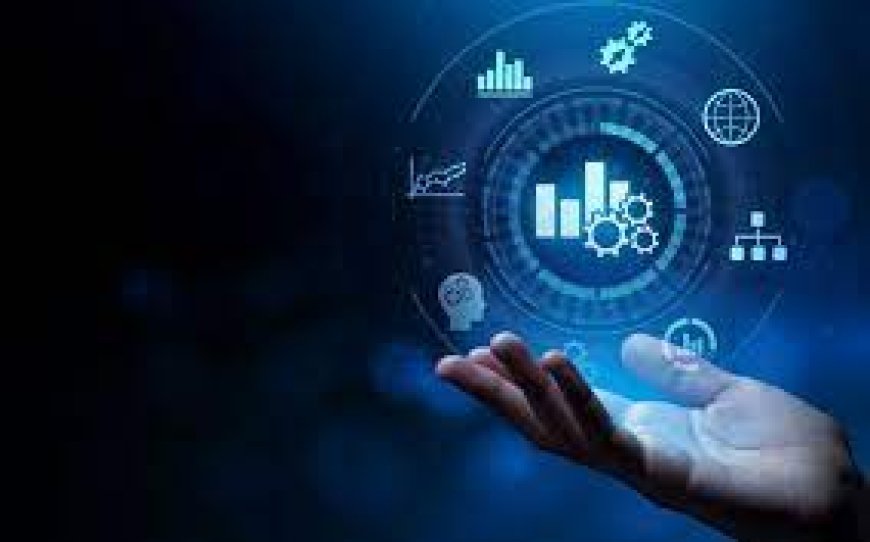
In today's data-driven world, businesses are increasingly turning to big data analytics solutions to gain insights that drive predictive analytics and forecasting. This blog explores how organizations can leverage these solutions effectively, focusing on key concepts, benefits, and best practices.
Understanding Predictive Analytics and Forecasting
Predictive analytics involves using historical data, statistical algorithms, and machine learning techniques to forecast future outcomes. By analyzing patterns and trends in data, organizations can make informed predictions about future events or behaviors. Forecasting, on the other hand, uses past data to estimate future trends and patterns, helping businesses anticipate market demands, customer behavior, and operational needs.
Key Components of Big Data Analytics Solutions
Big data analytics solutions for predictive analytics and forecasting typically include the following components:
1. Data Collection and Integration: Gathering data from multiple sources, including transactional databases, social media platforms, IoT devices, and more. Integration ensures all relevant data is consolidated for analysis.
2. Data Storage and Management: Storing large volumes of structured and unstructured data in scalable storage solutions, such as data lakes or cloud-based storage. Efficient data management ensures data accessibility and reliability.
3. Data Processing and Analysis: Utilizing advanced analytics techniques, including statistical analysis, machine learning, and AI algorithms, to uncover patterns, correlations, and anomalies in the data. This step forms the basis for predictive modeling and forecasting.
4. Visualization and Reporting: Presenting insights and predictions through visualizations such as charts, graphs, and dashboards. Clear visual representations help stakeholders understand complex data and make data-driven decisions.
Benefits of Big Data Analytics Solutions for Predictive Analytics and Forecasting
Implementing robust big data analytics solutions for predictive analytics and forecasting offers several advantages:
- Improved Decision Making: Predictive insights enable proactive decision-making based on anticipated future trends and outcomes.
- Enhanced Efficiency: Automating data analysis processes reduces manual effort and accelerates time-to-insight.
- Better Resource Allocation: Forecasting helps optimize resource allocation, such as inventory management, workforce planning, and budgeting.
- Competitive Advantage: Anticipating market trends and customer preferences allows businesses to stay ahead of competitors.
- Risk Mitigation: Identifying potential risks and threats enables preemptive measures to mitigate their impact.
Best Practices for Implementing Big Data Analytics Solutions
1. Define Clear Objectives and Use Cases
Before implementing a big data analytics solution for predictive analytics, define specific business objectives and use cases. Understand the problem you're trying to solve or the outcomes you aim to achieve through predictive insights.
2. Choose the Right Data Sources
Identify and integrate relevant data sources that provide valuable insights for predictive modeling. This may include transactional data, customer data, market data, social media data, and more. Ensure data quality and consistency to enhance the accuracy of predictions.
3. Select Suitable Algorithms and Models
Select appropriate machine learning algorithms and predictive models based on your data characteristics and business objectives. Common techniques include regression analysis, decision trees, neural networks, and time series forecasting models.
4. Ensure Scalability and Flexibility
Choose a scalable big data analytics solution that can accommodate growing data volumes and evolving business needs. Cloud-based solutions often offer scalability advantages, allowing you to expand resources as needed without infrastructure constraints.
5. Emphasize Data Security and Compliance
Implement robust security measures to protect sensitive data used in predictive analytics. Ensure compliance with data protection regulations, such as GDPR, especially when handling personal or ESG reporting tools.
6. Validate and Iterate
Validate predictive models using historical data and measure their accuracy against real-world outcomes. Continuously iterate and refine models based on new data and changing business conditions to improve predictive performance over time.
Case Study: Predictive Analytics in ESG Reporting
ESG (Environmental, Social, and Governance) reporting requires businesses to measure and report their sustainability performance. Big data analytics solutions can analyze esg reporting tools, such as energy consumption, carbon emissions, social impact metrics, and governance practices. Predictive analytics helps forecast future ESG trends, identify areas for improvement, and support strategic decision-making to achieve sustainability goals.
Conclusion
Big data analytics solutions empower organizations to leverage predictive analytics and forecasting for strategic advantage. By harnessing the power of data, businesses can anticipate market shifts, optimize operations, and enhance decision-making capabilities. Implementing these solutions requires careful planning, from defining objectives and selecting data sources to choosing algorithms and ensuring data security. Embrace the transformative potential of big data analytics to drive innovation, sustainability, and growth in today's competitive landscape.