The Transformative Power of Data Analytics: A Comprehensive Overview
Data analytics has fundamentally changed how businesses and organizations function in today's world. With the vast amounts of data generated daily, the ability to analyze and interpret this data has become a crucial asset.
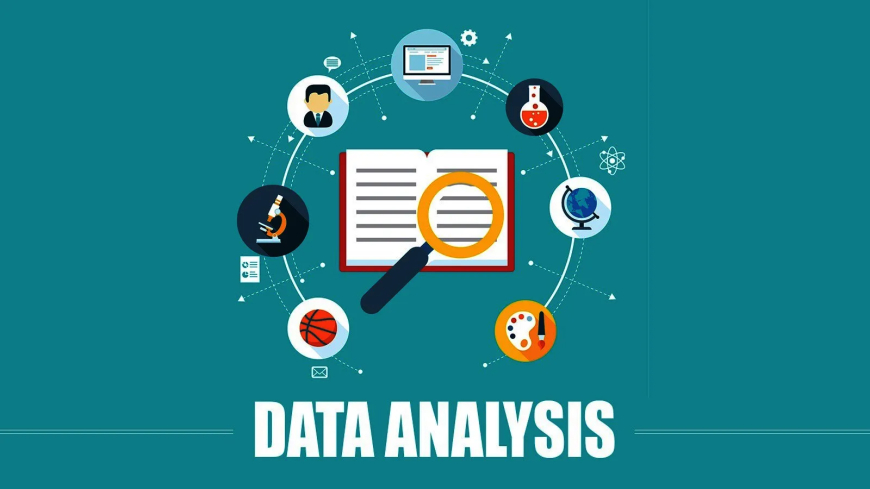
Data analytics has fundamentally changed how businesses and organizations function in today's world. With the vast amounts of data generated daily, the ability to analyze and interpret this data has become a crucial asset. From enhancing decision-making processes to uncovering hidden patterns, data analytics provides profound insights that drive efficiency, innovation, and competitive advantage. This article explores the multifaceted world of data analytics, examining its key components, methodologies, applications, and future trends.
Understanding Data Analytics
Data analytics involves examining raw data to draw meaningful conclusions. This process encompasses various techniques and tools designed to handle data in its structured, semi-structured, and unstructured forms. The primary goal of data analytics is to extract actionable insights from data, helping organizations make informed decisions.
Types of Data Analytics
-
Descriptive Analytics: Focuses on condensing historical information to comprehend historical occurrences. Data mining and data aggregation are popular methods.
-
Diagnostic Analytics: Determines why something happened, using advanced techniques like drill-down, data discovery, and correlations.
-
Predictive Analytics: Forecasts future trends based on historical data using machine learning, statistical modeling, and neural networks.
-
Prescriptive Analytics: Suggests actions to take for optimal outcomes using complex algorithms and simulations.
Key Components of Data Analytics
Data Collection
Data gathering is the initial stage of any data analytics process. This involves gathering data from various sources, including databases, data lakes, and external data streams. Ensuring the data's quality and relevance is crucial at this stage.
Data Cleaning
Raw data often contains inconsistencies, missing values, and errors. Data cleaning is the process of correcting or removing these inaccuracies to ensure high-quality data. Techniques like data imputation, normalization, and deduplication are commonly used.
Data Analysis
Once the data is cleaned, it undergoes analysis. This stage employs various statistical and computational methods to uncover patterns, correlations, and trends. Tools like SQL, Python, R, and specialized analytics software play a vital role in this phase.
Data Visualization
Presenting data in a comprehensible manner is critical. To successfully convey insights, data visualization entails graphical data representations. Tools like Tableau, Power BI, and D3.js are popular for their robust visualization capabilities.
Applications of Data Analytics
Business Intelligence
Businesses use data analytics to gain a competitive edge. Through business intelligence (BI) tools, companies can monitor performance metrics, identify market trends, and make strategic decisions. For instance, retailers use BI to optimize inventory levels and personalize marketing campaigns.
Healthcare
Data analytics is essential to improve patient outcomes in the healthcare industry. Predictive analytics can forecast disease outbreaks, while diagnostic analytics help in identifying effective treatment plans. Electronic Health Records (EHRs) and wearable devices generate valuable data that can be analyzed to enhance patient care.
Finance
Data analytics is a key component of the banking industry's risk management, fraud detection, and investing strategies. By analyzing transaction data, financial institutions can identify suspicious activities and mitigate risks. Making well-informed investing decisions is aided by predictive models.
Marketing
Marketers leverage data analytics to understand customer behavior and preferences. By analyzing social media interactions, purchase history, and browsing patterns, companies can create targeted marketing campaigns. Higher engagement and conversion rates result from this.
Manufacturing
In manufacturing, data analytics is used to optimize production processes, reduce downtime, and improve product quality. Predictive maintenance, driven by data analytics, can foresee equipment failures and schedule timely repairs, saving costs and preventing production halts.
Methodologies in Data Analytics
Machine Learning
A branch of artificial intelligence called machine learning (ML) gives systems the ability to learn from data and get better over time. Supervised, unsupervised, and reinforcement learning are key ML techniques used in data analytics. Applications range from predictive maintenance to customer segmentation.
Statistical Analysis
Statistical methods are foundational in data analytics. Techniques such as regression analysis, hypothesis testing, and Bayesian inference are employed to interpret data and draw conclusions. These methods are essential for validating the insights derived from data.
Big Data Technologies
Handling large volumes of data requires specialized technologies. Big data tools like Hadoop, Spark, and NoSQL databases enable the processing and analysis of massive datasets. These technologies support real-time analytics and are crucial for industries dealing with vast amounts of data.
Challenges in Data Analytics
Despite its potential, data analytics faces several challenges:
-
Data Privacy and Security: With the increasing amount of data being collected, ensuring its privacy and security is paramount. Regulations such as GDPR require organizations to put strong security measures in place.
-
Data Quality: Inaccurate insights might result from low-quality data. Ensuring data accuracy, completeness, and consistency is a continuous challenge.
-
Skill Gap: The demand for skilled data analysts and scientists often exceeds supply. Bridging this skill gap through education and training is essential for leveraging data analytics effectively.
-
Integration: It might be challenging to integrate data from several sources. Organizations need to ensure seamless data integration to create a unified view of their data.
Future Trends in Data Analytics
Artificial Intelligence and Machine Learning
AI and ML will continue to drive advancements in data analytics. As these technologies evolve, they will offer more sophisticated tools for predictive and prescriptive analytics. Automated machine learning (AutoML) is expected to simplify model building and deployment.
Edge Analytics
Processing data closer to its source at the network's edge is known as edge analytics. This is perfect for Internet of Things applications since it lowers latency and bandwidth utilization. Edge analytics will enable real-time decision-making in sectors like manufacturing and healthcare.
Augmented Analytics
AI and ML are used in augmented analytics to improve data preparation, analysis, and visualization. By automating complex tasks, augmented analytics allows users to focus on interpreting insights and making data-driven decisions.
Data Democratization
Data democratization aims to make data accessible to non-technical users. With user-friendly tools and platforms, employees across an organization can analyze data without needing specialized skills. This trend will empower more people to leverage data in their roles.
Conclusion
Data analytics has become an indispensable tool in today's data-driven world. Its ability to transform raw data into actionable insights empowers organizations to make better decisions, optimize operations, and innovate continuously. As technology advances, the future of data analytics promises even greater capabilities, driving further growth and transformation across industries. Embracing data analytics, perhaps through a Data Analytics course in Thane, Mumbai, Navi Mumbai, Delhi, Noida and other cities of India is not just a competitive advantage; it is a necessity for staying relevant and thriving in the modern landscape.