The Evolution of Data Analytics: Past, Present, and Future
The evolution of data analytics spans from manual processes to AI-driven insights, transforming industries by enabling data-driven decision-making. Key developments include the rise of big data, machine learning, real-time analytics, and emerging technologies like quantum computing. With growing demand, professionals skilled in analytics are essential for future business success.
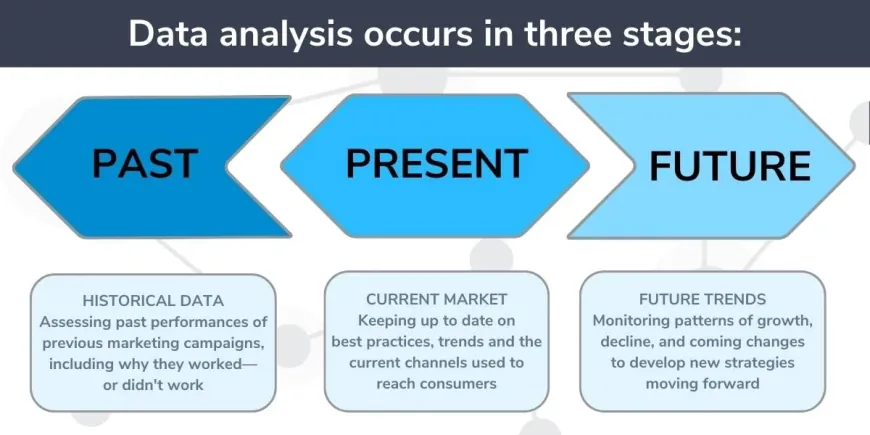
In today’s technology-driven world, data analytics has become indispensable for decision-making across industries like healthcare, finance, marketing, and e-commerce. The evolution of data analytics has been remarkable, transitioning from simple manual calculations to sophisticated AI and machine learning-driven systems capable of real-time analysis. This transformation has reshaped how organizations collect, process, and utilize data while also creating a demand for professionals skilled in extracting valuable insights from vast datasets.
The Past: The Foundations of Data Analytics
The roots of data analytics can be traced back centuries, where mathematicians and scientists sought ways to interpret numerical data. However, the modern era of data analytics took shape in the mid-20th century, with the rise of computers and more advanced technology.
1. Pre-Digital Era: The Manual Approach
Before the digital age, data analysis was performed manually. Statisticians and analysts gathered data through surveys, observations, and experiments, then analyzed it using basic statistical tools. Methods were limited to descriptive statistics, which helped organizations understand past performance but offered no predictive insights. Tools like pen-and-paper, mechanical calculators, and early electronic devices assisted in these calculations. Businesses typically relied on intuitive decision-making backed by trends and reports, which were slow to produce.
2. The Mainframe Era (1950s - 1970s)
The introduction of mainframe computers in the mid-20th century marked a significant leap in data processing. Companies such as IBM developed systems that could handle larger datasets, enabling businesses to store, retrieve, and process information more efficiently. Early data analytics focused on structured data, such as transactional records and financial statements.
During this time, Management Information Systems (MIS) emerged, which allowed for centralized data storage and the generation of regular reports. However, the analysis remained slow and required specialized skills, as the process involved batch processing with limited real-time capabilities.
3. The Rise of Relational Databases (1970s - 1980s)
The development of relational databases in the 1970s, pioneered by Edgar F. Codd, revolutionized data storage and accessibility. By organizing data into tables that could be linked by shared fields, relational databases made querying data easier and faster. SQL (Structured Query Language) became the standard for database management, providing a powerful tool for querying, analyzing, and managing data.
This era also saw the rise of business intelligence (BI) tools, which enabled organizations to generate more detailed reports and analyze historical data. These tools laid the foundation for more advanced analytics techniques by allowing businesses to slice and dice their data in more meaningful ways.
The Present: Big Data and Real-Time Analytics
Over the past two decades, data analytics has entered a new era, driven by the explosion of data generation, often referred to as "Big Data." Today, data is produced from numerous sources—social media, sensors, IoT devices, and digital transactions—creating a wealth of information to be analyzed.
1. The Big Data Era (2000s)
The rise of Big Data has dramatically changed the data analytics landscape. Traditional systems could no longer cope with the scale, variety, and speed of modern data. Technologies like Hadoop and Apache Spark emerged to handle large datasets and process both structured and unstructured data. These innovations paved the way for more advanced analysis, enabling businesses to predict trends, identify patterns, and make data-driven decisions.
Predictive analytics gained prominence during this period, allowing organizations to forecast future trends based on historical data. This shift had a profound impact across industries. For example, retailers began predicting customer demand, while finance and healthcare industries leveraged predictive analytics for risk assessment and patient care, respectively.
2. Artificial Intelligence and Machine Learning (2010s)
The 2010s saw the rapid advancement of artificial intelligence (AI) and machine learning (ML), both of which have become integral to modern data analytics. These technologies enable systems to learn from data, discover patterns, and make decisions without explicit programming. In industries such as finance, e-commerce, and marketing, AI and ML are used to detect fraud, recommend products, and optimize customer engagement.
This era also saw the emergence of the data scientist, a professional skilled in mathematics, programming, and domain expertise. Data scientists design algorithms, build predictive models, and interpret data, playing a critical role in transforming raw data into actionable insights. AI-driven models shifted the focus from merely understanding historical data to forecasting future events and automating decision-making processes.
3. Real-Time Analytics and the Cloud
The growing capabilities of cloud computing have enabled businesses to analyze data in real time. Technologies like Apache Kafka and Amazon Kinesis make it possible to capture, process, and analyze massive amounts of data instantly. This is particularly valuable in sectors like finance, healthcare, and logistics, where timely decisions are essential.
Real-time analytics allows companies to monitor changing conditions and make immediate adjustments. In financial markets, real-time data enables traders to respond quickly to market fluctuations. In healthcare, real-time analytics helps monitor patient vitals and conditions, improving treatment outcomes.
The Future: Trends Shaping Data Analytics
As data analytics continues to evolve, several emerging trends and technologies will likely shape its future. These developments promise to further expand the capabilities of organizations to make smarter, faster, and more informed decisions.
1. Augmented Analytics
Augmented analytics is set to revolutionize the field by leveraging AI and ML to automate the process of data preparation, analysis, and reporting. This technology will democratize data analytics, making it accessible to non-technical professionals and reducing reliance on data scientists. Augmented analytics tools will help businesses derive insights more quickly and accurately by automating complex tasks.
As augmented analytics grows, more businesses will be able to adopt data-driven decision-making processes, fostering a culture of innovation and operational efficiency.
2. Edge Analytics
Edge computing, which processes data closer to its source (such as IoT devices or local servers), is expected to play a significant role in the future of data analytics. Edge analytics allows for faster, real-time analysis and decision-making in environments where quick responses are crucial. This is especially relevant in industries like manufacturing, transportation, and healthcare, where sensors and devices generate data that needs immediate processing.
With the rise of connected devices, edge analytics will enable organizations to gain insights directly at the data source, reducing latency and improving operational agility.
3. Ethical and Responsible Data Usage
As data continues to proliferate, the ethical considerations surrounding its use will become increasingly important. Regulations like GDPR and CCPA emphasize the need for organizations to handle personal data responsibly, ensuring transparency and user control. In the future, businesses will need to focus on ethical data practices, building trust with consumers, and preventing biases in AI-driven analytics.
Transparency in data collection, processing, and analytics will be critical, as well as the responsible use of AI models to ensure that decisions are fair and free from discrimination.
4. Quantum Computing
Quantum computing, though still in its infancy, holds immense potential for data analytics. Capable of processing vast amounts of information far faster than traditional computers, quantum computing could unlock new possibilities in areas like cryptography, climate modeling, and drug discovery. Once fully realized, quantum computing will enable businesses to analyze larger datasets with greater speed and precision, tackling problems that are currently unsolvable.
Conclusion
The evolution of data analytics—from manual processes to AI-driven insights—has been transformative for businesses worldwide. Today, organizations rely on advanced analytics to predict trends, improve efficiency, and gain competitive advantages. Looking ahead, emerging technologies such as augmented analytics, edge computing, and quantum computing will continue to drive innovation in the field, making data more accessible and actionable than ever before.
As the demand for data-driven insights continues to grow, professionals skilled in analytics will remain essential to the success of businesses across all industries. To meet this growing demand, institutes like Uncodemy offer the best Data Analytics course in Delhi, Noida, Gurugram, Mumbai, Navi Mumbai, Thane, and other locations across India, providing comprehensive training to equip individuals with the latest tools and techniques. These courses ensure that learners are prepared to navigate the future of this exciting field and become proficient in the skills that modern businesses need to thrive.
With the continued rise of data-driven strategies, professionals well-versed in analytics will play a pivotal role in shaping the future of industries across the globe.