MLOps Market: Transforming the Future of Machine Learning Operations (2025-2034)
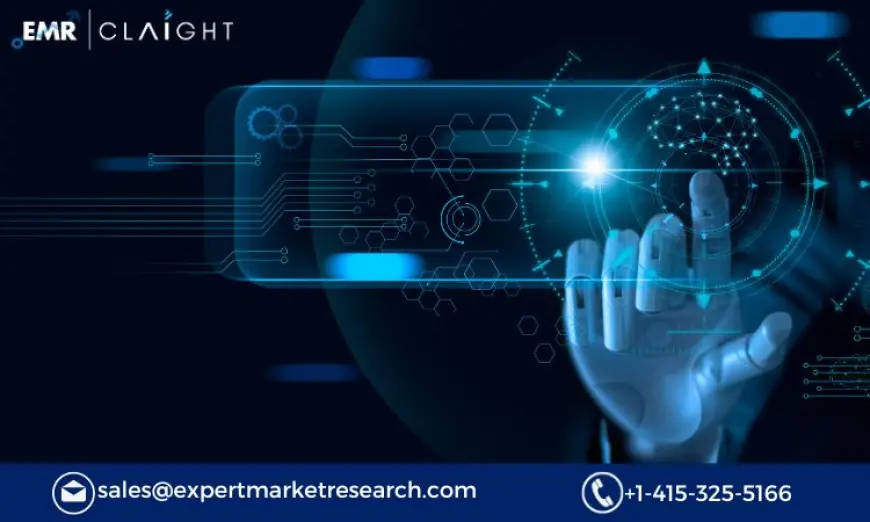
The global MLOps market size reached around USD 4.26 billion in 2024. The market is projected to grow at a CAGR of 32.70% between 2025 and 2034 to reach nearly USD 72.13 billion by 2034. This extraordinary growth underscores the increasing importance of machine learning operations (MLOps) in managing the lifecycle of AI models. As industries worldwide leverage artificial intelligence (AI) and machine learning (ML) to streamline operations, the demand for efficient, scalable, and secure platforms to manage these models is surging. In this blog post, we will explore the key trends, market drivers, challenges, and opportunities in the MLOps market, providing an in-depth look at its growth trajectory and the factors shaping its future.
Market Overview
MLOps, or Machine Learning Operations, is an emerging field designed to bring the benefits of DevOps practices to the world of machine learning. It integrates various aspects of machine learning workflows—from model development and training to deployment, monitoring, and governance—into a streamlined, automated pipeline. MLOps plays a critical role in managing complex machine learning models by ensuring their scalability, reliability, and continuous optimization.
The global MLOps market is evolving rapidly as businesses seek to scale their machine learning operations and implement automated, seamless workflows. With more organizations adopting AI technologies, MLOps solutions are becoming essential for managing large volumes of data and ensuring that machine learning models deliver accurate, timely, and actionable insights.
Market Segmentation
By Component
Platform
The platform component of the MLOps market includes solutions that facilitate the management of the machine learning lifecycle, from data preparation and model building to deployment and monitoring. These platforms provide key features such as version control, automated model training, deployment management, and model monitoring. Leading platforms in the MLOps space, such as Amazon Web Services (AWS) SageMaker, Microsoft Azure Machine Learning, Google Cloud AI, and open-source platforms like Kubeflow, enable enterprises to manage machine learning workflows effectively.
Service
MLOps services are integral to helping organizations implement MLOps practices and ensure seamless integration with existing infrastructure. These services typically include consulting, system integration, managed services, and support for scaling machine learning models across large operations. As MLOps becomes more complex, organizations are turning to specialized service providers to help them implement best practices, manage compliance requirements, and drive business outcomes.
By Deployment Mode
On-Premise
On-premise deployment of MLOps solutions is often preferred by organizations that require complete control over their data and infrastructure. While on-premise solutions offer high security and customization, they also come with the challenge of requiring substantial upfront investment in hardware and software. Large enterprises in industries such as banking and government prefer on-premise deployment due to the sensitive nature of their data.
Cloud
Cloud-based MLOps solutions have gained widespread adoption due to their scalability, flexibility, and cost-effectiveness. Cloud infrastructure provides organizations with the ability to scale their AI models quickly without investing heavily in physical infrastructure. Major cloud providers such as AWS, Microsoft Azure, and Google Cloud offer MLOps tools as part of their cloud-based AI/ML services, enabling organizations to deploy, monitor, and optimize their machine learning models efficiently.
By Organization Size
Large Enterprises
Large enterprises are the primary consumers of MLOps solutions, owing to their significant investments in machine learning and AI initiatives. These enterprises often have complex, multi-regional operations and require robust, scalable MLOps platforms that can handle large volumes of data and numerous machine learning models. The ability to manage models at scale and ensure consistent performance across the organization is a top priority for these businesses.
Small and Medium-sized Enterprises (SMEs)
While large enterprises lead the adoption of MLOps, SMEs are increasingly exploring these solutions to boost their AI capabilities. Cloud-based MLOps platforms, in particular, offer cost-effective options for SMEs that are looking to automate and scale their machine learning processes without the need for extensive infrastructure investments. The ability to access enterprise-grade MLOps tools without a large upfront investment is enabling SMEs to capitalize on AI and machine learning technologies.
By Industry Vertical
BFSI (Banking, Financial Services, and Insurance)
The BFSI sector is one of the largest adopters of MLOps, using machine learning for a range of applications, including fraud detection, risk management, personalized financial services, and customer segmentation. The need for robust monitoring and compliance in these applications has made MLOps an essential part of their AI operations. MLOps ensures that machine learning models in the BFSI sector are continuously updated and optimized for accuracy and compliance.
Manufacturing
In manufacturing, MLOps is increasingly being used to drive operational efficiencies through predictive maintenance, supply chain optimization, and quality control. Machine learning models help predict equipment failures before they happen, improving asset management and reducing downtime. MLOps facilitates the deployment and management of these models, ensuring that they remain effective as manufacturing processes evolve.
IT and Telecom
The IT and telecom industries leverage MLOps to enhance customer experience, optimize networks, and streamline operations. Machine learning models are used for applications such as predictive network maintenance, fraud detection, and customer behavior analysis. As these industries rely heavily on large datasets and real-time analytics, MLOps ensures that models can be deployed quickly and monitored continuously for optimal performance.
Retail and E-commerce
In the retail and e-commerce sectors, MLOps enables businesses to deliver personalized recommendations, optimize inventory, and predict customer demand. The ability to deploy machine learning models in real-time is critical for maintaining a competitive edge in a fast-paced market. MLOps tools automate the deployment and scaling of models, making it easier for retailers to improve customer engagement and operational efficiency.
Healthcare
Healthcare organizations are increasingly using machine learning models to analyze medical images, predict disease outbreaks, and develop personalized treatment plans. MLOps helps ensure that these models are continuously updated and optimized, providing clinicians with the most accurate and timely insights. Given the sensitive nature of healthcare data, MLOps solutions in this industry must comply with strict data privacy regulations.
Other Sectors
Other industries, including energy, utilities, logistics, and automotive, are also adopting MLOps to drive efficiencies and innovation. For example, in the energy sector, MLOps solutions are used to optimize the distribution of energy, while in the automotive industry, machine learning models power autonomous vehicles and predictive maintenance.
Market Dynamics
Key Drivers of Market Growth
- Rising Adoption of AI/ML: As more industries embrace AI and machine learning, the demand for MLOps solutions to manage these models is increasing. MLOps helps automate processes, improve accuracy, and scale machine learning applications.
- Operational Efficiency: MLOps enables businesses to streamline their machine learning workflows, reducing manual intervention and improving productivity. By automating model deployment and monitoring, organizations can save time and resources.
- Need for Continuous Model Optimization: Machine learning models require constant monitoring and optimization to maintain accuracy and relevance. MLOps solutions provide the tools needed to ensure that models remain effective in dynamic environments.
- Cloud Adoption: The rapid adoption of cloud computing has made it easier for organizations to scale their machine learning operations. Cloud-based MLOps platforms offer flexibility and cost savings, driving their widespread use.
Market Challenges
- Complexity of MLOps Solutions: Integrating MLOps tools with existing IT infrastructure can be complex, especially for organizations that lack the necessary expertise. The learning curve associated with MLOps adoption can be a barrier to entry for some businesses.
- Data Privacy and Security Concerns: Ensuring the security of data and compliance with privacy regulations is crucial for industries like healthcare and finance. MLOps solutions must provide robust security features to address these concerns.
- Lack of Standardization: The MLOps market is still evolving, and a lack of standardization in practices and tools can lead to interoperability issues and challenges in integrating different components of the machine learning pipeline.
Competitive Landscape
The MLOps market is highly competitive, with major players such as Microsoft, Google, Amazon Web Services (AWS), IBM, and Databricks leading the charge. These companies offer comprehensive MLOps platforms that include model development, deployment, monitoring, and management tools. Startups and niche players are also emerging, providing specialized solutions tailored to specific industries or use cases. The key strategies adopted by market leaders include product innovation, strategic partnerships, and expanding cloud-based service offerings to cater to a broader range of customers.